Stock market prediction AI involves the use of artificial intelligence (AI) and machine learning (ML) techniques to forecast the future movements of stock prices. By analyzing vast amounts of historical data and current market trends, AI can identify patterns and make predictions that can help investors make informed decisions.
Table of Contents
Importance of Stock Market Prediction AI
With the increasing complexity of financial markets and the sheer volume of data generated every second, traditional methods of stock market analysis are becoming less effective. AI offers a more sophisticated approach by processing large datasets and identifying non-obvious patterns that human analysts might miss. This has the potential to revolutionize investment strategies and improve market efficiency.
Understanding Stock Market Prediction AI
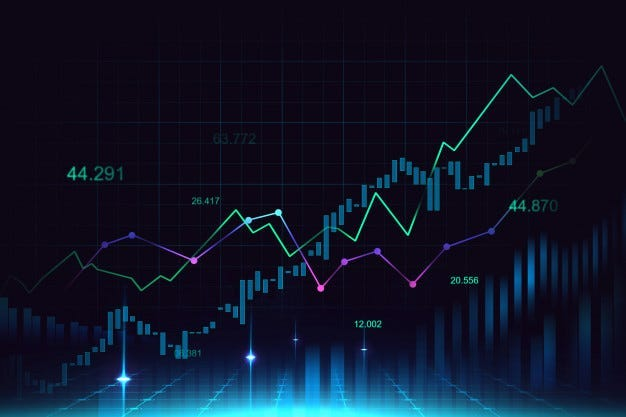
Stock market prediction AI combines various AI technologies, including neural networks, natural language processing (NLP), and deep learning, to predict stock price movements. These technologies enable the AI to analyze historical price data, financial reports, news articles, and social media sentiments.
How it Works
- Data Collection: Gathering historical stock prices, financial statements, economic indicators, news, and social media data.
- Data Preprocessing: Cleaning and normalizing the data to ensure accuracy and consistency.
- Feature Engineering: Extracting relevant features such as moving averages, trading volumes, and sentiment scores.
- Model Training: Using machine learning algorithms to train models on historical data.
- Model Evaluation: Testing the models on unseen data to evaluate their predictive accuracy.
- Prediction: Using the trained models to make future predictions.
Key Components of Stock Market Prediction AI
- Data Collection: The quality and quantity of data play a crucial role in the accuracy of predictions.
- Feature Engineering: Identifying the right features that influence stock prices is critical for model performance.
- Model Selection: Different machine learning models have different strengths; selecting the right one is essential.
- Training and Tuning: Properly training and fine-tuning the model is necessary for achieving high accuracy.
The Role of Machine Learning in Stock Market Prediction
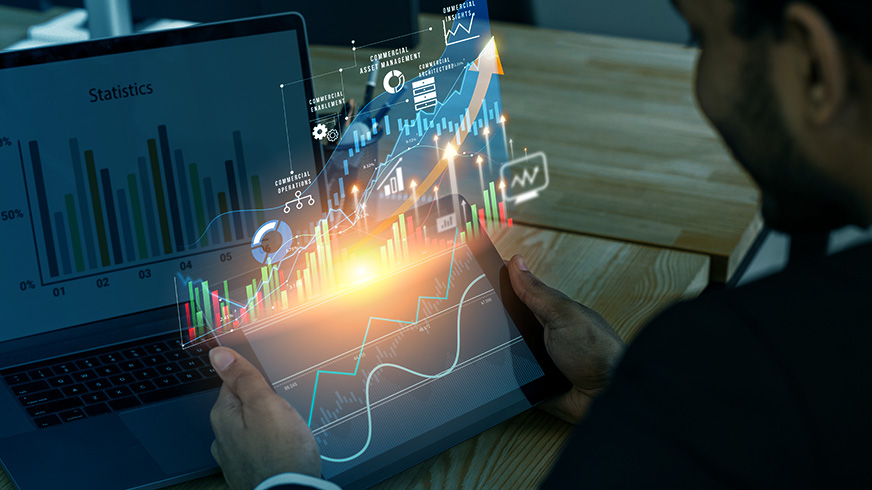
Types of Machine Learning Models
Supervised Learning: Models are trained on labeled historical data to predict future stock prices.Unsupervised Learning: Models identify patterns in data without pre-labeled outcomes, useful for clustering similar stocks.Reinforcement Learning: Models learn to make trading decisions by interacting with the market environment and receiving feedback.
Popular Algorithms
- Linear Regression: Predicts stock prices based on historical price trends.
- Decision Trees: Models decision rules to predict future stock movements.
- Random Forest: An ensemble of decision trees for better accuracy.
- Support Vector Machines (SVM): Classifies stock price movements by finding the optimal boundary.
- Neural Networks: Deep learning models that can capture complex relationships in data.
Practical Applications of Stock Market Prediction AI
Algorithmic Trading
Algorithmic trading involves using AI to execute trades based on predefined criteria. AI algorithms can make split-second trading decisions, taking advantage of market inefficiencies and executing high-frequency trades.
Portfolio Management
AI can help in creating and managing investment portfolios by predicting the performance of different stocks and optimizing asset allocation.
Risk Management
AI models can assess the risk associated with different investment strategies and help investors minimize potential losses.
Market Sentiment Analysis
By analyzing news articles, social media posts, and other textual data, AI can gauge market sentiment and predict how it might affect stock prices.
Challenges in Stock Market Prediction AI
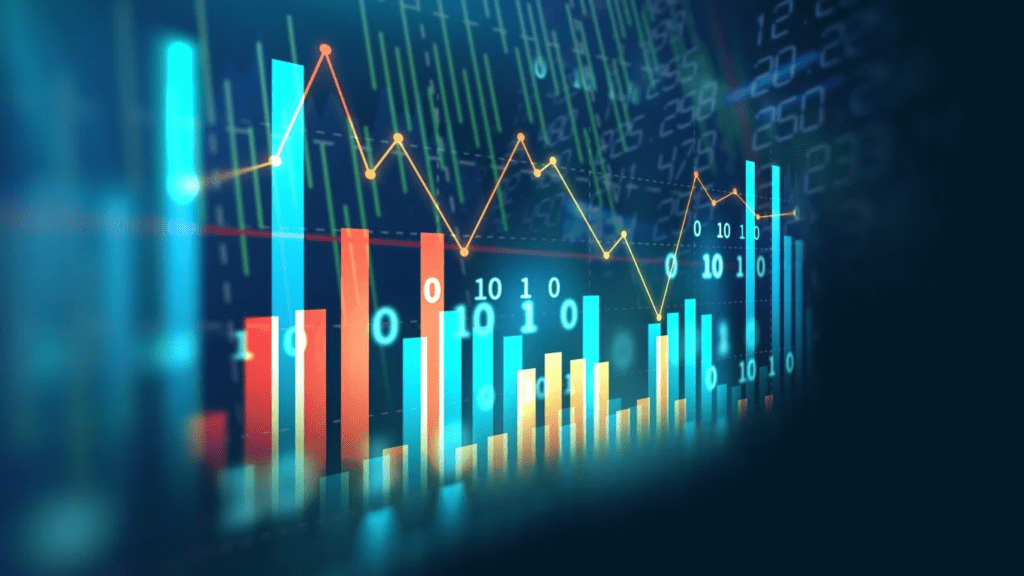
Data Quality and Availability
High-quality data is essential for accurate predictions. However, financial data can be noisy and incomplete, posing a challenge for AI models.
Market Volatility
Stock markets are influenced by numerous unpredictable factors, making accurate prediction difficult.
Overfitting
AI models can sometimes learn from noise in the data rather than the underlying patterns, leading to overfitting and poor predictive performance on new data.
Ethical and Regulatory Concerns
The use of AI in stock trading raises ethical and regulatory issues, including market manipulation and transparency.
How to Start a Blog on Stock Marketai stock market predictionPrediction AI
Identifying Your Target Audience
Determine who your readers will be – novice investors, experienced traders, or tech enthusiasts interested in AI.
Choosing a Blogging Platform
Select a platform that suits your needs, such as WordPress, Blogger, or Medium.
Creating Quality Content
- Educational Articles: Explain complex AI concepts in simple terms.
- Market Analysis: Provide insights and predictions based on AI models.
- Tutorials: Guide readers on how to use AI tools for stock market prediction.
- Case Studies: Share real-world applications and success stories.
Engaging with Your Audience
Respond to comments, engage in discussions, and use social media to connect with your readers.
Conclusion
Stock market prediction AI represents a powerful tool that can transform the way we approach investing. By leveraging advanced machine learning techniques, investors can gain deeper insights and make more informed decisions. Starting a blog on this topic not only allows you to share valuable knowledge but also positions you as an authority in this rapidly evolving field.
FAQs
Can AI predict stock prices accurately?
While AI can identify patterns and make predictions, it is not infallible. Market conditions can change rapidly, and AI models need continuous updates and validation.
What are the best AI algorithms for stock market prediction?
Popular algorithms include linear regression, decision trees, random forests, support vector machines, and neural networks. The choice depends on the specific application and data.
How can I start learning about stock market prediction AI?
Begin with online courses on AI and machine learning, read research papers, and experiment with AI tools on historical stock data.
Is AI-based trading ethical?
AI-based trading is ethical as long as it complies with regulatory guidelines and is transparent. However, it raises concerns about market fairness and the potential for manipulation.
How often should AI models be updated?
AI models should be updated regularly to incorporate new data and adapt to changing market conditions. The frequency of updates depends on the specific use case and market volatility.